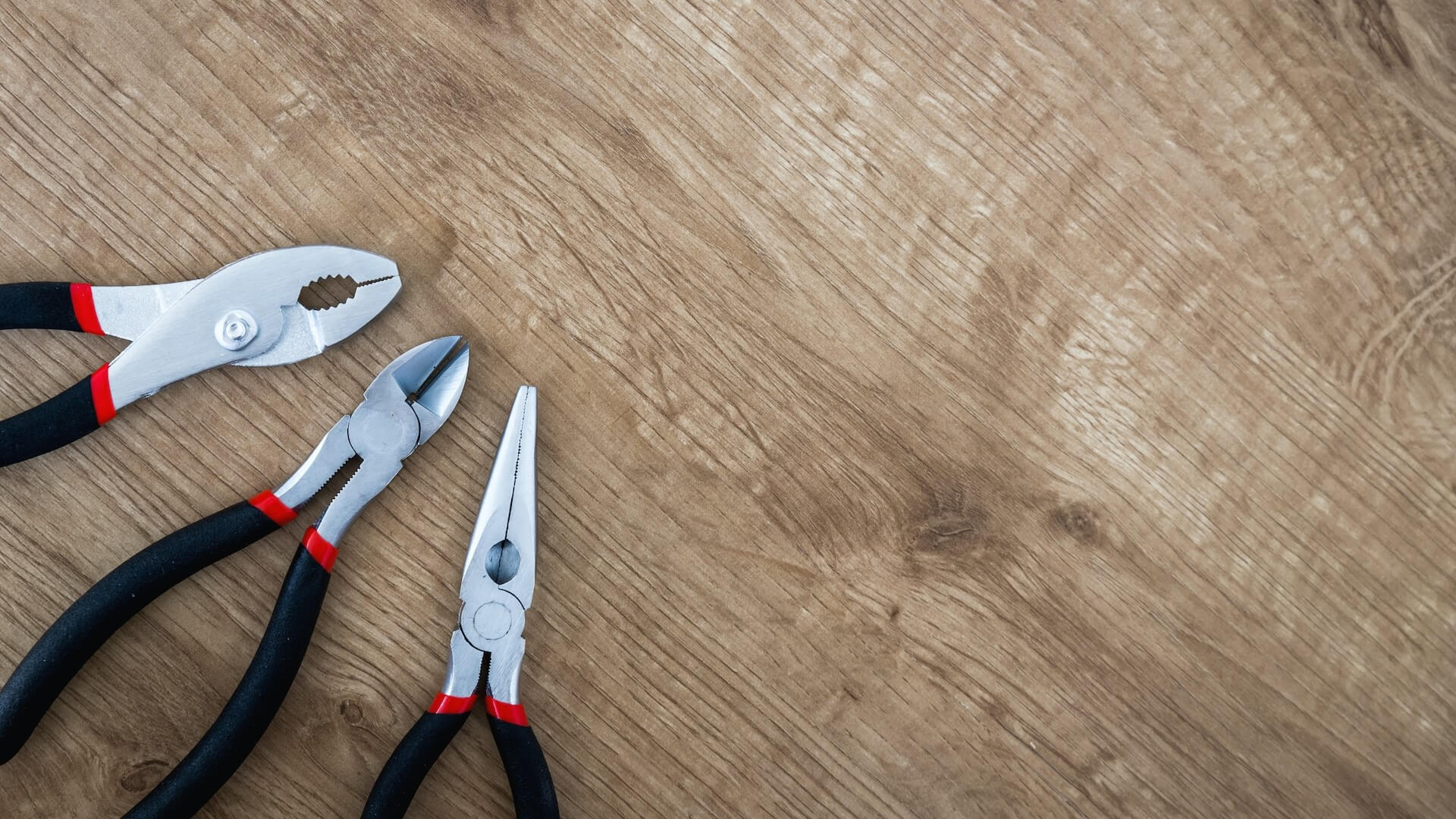
While the impact of IoT sensors is multifaceted, there may be nothing more important to modern companies than predictive maintenance tools. According to a report by Deloitte, predicting failures of corporate assets can increase equipment uptime by 20 percent, increase productivity by 25 percent, and reduce breakdowns by 70 percent.
On top of that, the study found that predictive maintenance can reduce maintenance costs by 25 percent. This could be a lifesaver for many industries, which begs the question, what is predictive maintenance and how does it work?
What is predictive maintenance?
The ultimate goal of predictive maintenance is to avoid costly downtime by successfully predicting when an asset will fail and performing maintenance only when it is needed. To do this, data collected by environmental sensors and other IoT monitoring devices needs to be thoroughly reviewed and analyzed to create actionable insights and usage patterns on the performance of mission-critical equipment.
By its very nature, predictive maintenance improves on reactive models where unplanned downtime is unavoidable. Organizations that adopt time-based maintenance plans may be able to avoid unplanned downtime, but the costs of inefficient asset maintenance can also add up quickly.
The primary risk is maintaining assets too frequently, resulting in unnecessary expenditures for replacing asset parts or equipment that are still serviceable. Monitoring these same assets and maintaining them on a more efficient schedule can save up to 12% over regular maintenance.
How does it work?
At its core, all predictive maintenance starts with monitoring specific conditions of the equipment. These conditions are typically based on historical performance data or equipment specifications and are designed to create a range for the optimal performance environment for the asset. This creates a monitoring mechanism to compare the current condition of each asset. These conditions are observed through IoT sensors, and the data is monitored for any abnormal behavior that could lead to potential failure.
There are a wide variety of sensors used in predictive maintenance, the most common of which are: temperature sensors, humidity sensors, motion sensors, sound sensors, light wave sensors, current sensors, etc.
Of course, simpler IoT solutions like security cameras are also an important part of predictive maintenance. Being able to observe any visible changes in an asset from a remote location is especially valuable for maintenance in use cases that span geographic areas, such as oil pipelines or power lines.
Artificial intelligence and IoT solutions
Of course, failures don't exactly occur during working hours, so relying on the human eye to monitor hundreds of potential predictive maintenance data streams is often not the most effective, so developers employ artificial intelligence to analyze unusual changes in asset performance.
Artificial intelligence typically extracts data from IoT sensors based on statistical models built from historical data, runs the data against parameters outlined as signs of potential degradation, and creates notifications when these conditions are met. To do this, AI creates mathematical models that encode factors such as temperature and activity into simple numerical points.
Scott Genzer, a data scientist at RapidMiner, says, "This is really nothing more than the old-fashioned mathematical model we've been doing for decades, the difference being that we now have the computational power to process large amounts of data to figure out patterns."